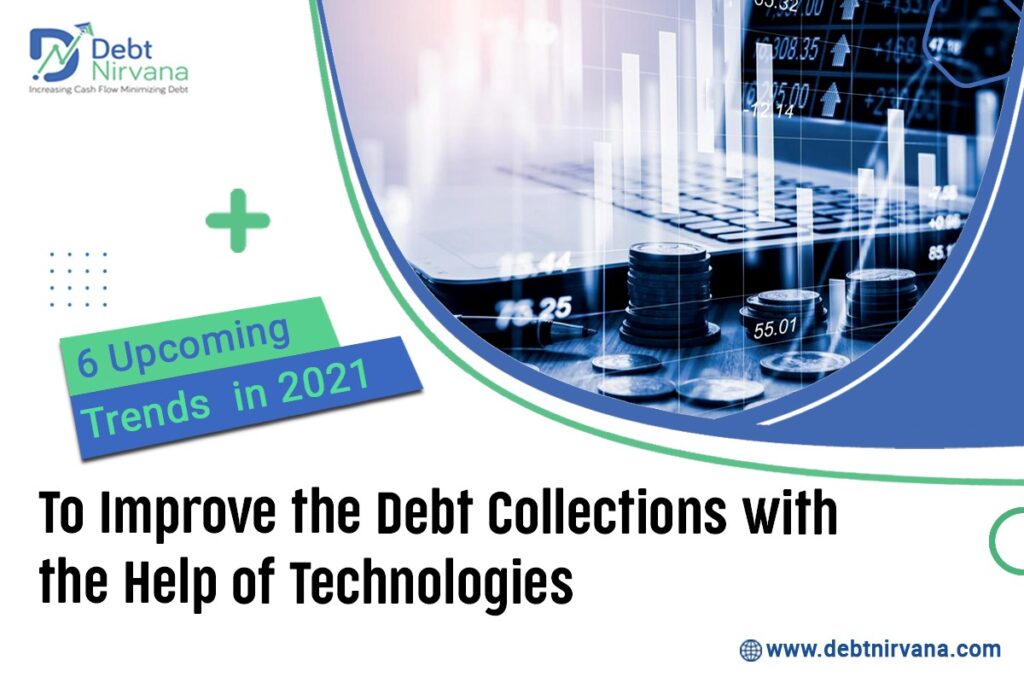

6 Upcoming Trends in 2024 to Improve the Debt Collections with the Help of MI (Machine Learning) and AI (Artificial Intelligence)
MI (Machine Learning) and AI (Artificial Intelligence) have shown to be the most attention-seeking technology by far, presenting their appearance in each industry. These technologies have been industry agnostic, decoding various problems by automating many of the significant methods and diminishing personal invasion.
It is comprehended that the initiation of the best technology to determine/optimise the dilemma increases the consumer experience and causes expertise in the system. ML/AI make utilisation of the open or generated dense info to induce out models to foretell the following steps. This eases small-size businesses to leverage data and balance much quicker, including such technological solutions as a component of their product offering.
One such economic industry product is lending. The transformation in the economic service quarter in the preceding couple of decades has been mind-boggling with the application of exceptional technology. Banks and commercial institutions raise various kinds of loans out of the funds raised from the saving account contributors and the funds borrowed from institutional investors. The range in the interest rates of those two stakeholders makes the Bank/Lending company viable. The significant dilemma is the management of unpaid loan accounts, like loan accounts where the borrowers have missed on their payments for a duration.
Currently, few platforms use Machine Learning, Artificial Intelligence resulting in the prophecy of the return opportunity for a delinquent borrower, based on various possible data points.
The 6 Upcoming Trends in 2024 to boost the Debt Collection with the help of technologies comprises:
1. Early Warning Signal:
Current analysis has exposed that one of the main causes for accounts converting delinquents is the lending companies’ incapacity to recognise stressed accounts that are expected to default. Numerous countries have rule-based monitoring methods for such early warning signals over profit identification, credit quality, and drift in the utilisation of reserves. An exceptional early warning system based on Artificial Intelligence practising openly available friendly activity data and knowledge on borrowers, blended with the above economic knowledge, will alleviate the uncertainty of accounts becoming delinquent. Machine Learning tools like Predictive Analytics, NLP, and Clustering will boost signals on such accounts.
2. Intent Identification and Yielding Validator:
Proposals made by agencies will be traced by ML speech-to-text models to recognise the fault of agencies. This will support economic institutions in satisfying compliance demands. Intent identification is a secondary application case, where based on the audio discussion of the Debt collection agency India and borrower, the tool can support recognising users with an unwillingness to settle.
3. Prompt Automation:
The usage of Machine Learning and Artificial Intelligence will support automating timelines to a follow-up call, content and time of the date based on prior data used to train alike borrowers.
4. Digital Communication Journey/Suggestions:
Currently moneylenders have been conveying everyday reminders with almost related content through all the ways, without any facts in place. The digital communication journey can be composed for managing innovation, while communication channels deliver performance in the development strategy. For instance, a borrower has opened the WhatsApp message 5 times out of 7 messages sent vs 0 intercommunication over email, the platform allows the subsequent set of contact to that borrower on WhatsApp, including conveying payment links over the chat with agencies.
5. Automatic Voice Bots (Robo Calling with IVR functions):
Automatic call bots will support a growing customer coverage rate with speech-to-text based figures to convey preceding automatic personalised and contextual communications with the borrowers, therefore lessening the chore of standard follow-ups and are cost-effective results. Convention call flows can be devised based on the usual conversation trends in an agency–borrower communication in person – like calls, in various dialects.
6. Recovery Risk Predictor:
ML-based Recovery Risk Predictor design based on various accumulations, EMI instalment, and geographic knowledge help prioritize/deprioritize the records for loan renewal – for instance, an account with a 70% possibility of giving back vs an account with a 30% probability. An account with a bigger possibility would be prioritised for collecting—lower price and time but the greater profit on the money paid for recovering. With the accounts on the lower surface of renewal possibility (usually on higher dpd), constitutional methods could have been chosen for such borrowers.
Summing it up, Globally, banks require to shift from a frequently compliance-driven post-facto method to a proactive digital structure centred on charges to follow credit risk. Debt accumulation has been the crucial component of each lending company and excellent technologies like AI and ML-powered designs can support the print real-time classification of stressed accounts, boost resolution, and automate conversation issues, thereby diminishing critical debts. As the information remains to get densely populated, more exceptional models will continue unfolding in this area to optimize the debt collecting methods.
If you are interested in such informative knowledge or looking for a Debt collection services, please get in touch with our expert team at Debt Nirvana.